Over the next two weeks, Headlight is publishing a three part series breaking down one of the ways we’re incorporating emergent AI technology to solve problems and improve marketing efficiency across our org. Large Language Models have been transformative in our ability to rapidly process and analyze large swaths of text. In part 1, we outline how we’re leveraging this new tool to help solve a core competitive intelligence need for our client partners. Subscribe to our blog to stay informed.
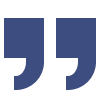
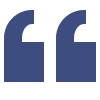
Customer journeys are becoming increasingly complex and nuanced. The pace of change is accelerating with technology acting as a catalyst to rapidly change customer expectations and needs. The upshot is misconceptions about your customers are now more costly than ever.
At Headlight, we have begun using Large Language Models (LLMs) like ChatGPT to identify emerging trends in consumer sentiment and needs for our client partners. This unlocks valuable insights that can drive product innovation, new testing and marketing decisions.
The Problem
Customer needs are changing faster than ever. How well do you really know your customers?
A prerequisite for effective marketing is to align your offering with your customer’s needs. A common underlying problem that many experienced marketers face is holding a fixed or oversimplified, one-dimensional view of customers. A 2023 study by Salesforce found “65% of customers expect companies to adapt to their changing needs and preferences, but 61% of customers say most companies treat them as a number.” Moreover, “80% of customers now value the experience a company provides as important as its products”. These findings underscore the critical importance of staying in lock-step with ever-evolving target customer needs and expectations. Tools like market testing, surveys and listening to feedback can help though real world feedback remains invaluable. By adapting to these changes, businesses can more easily align creative, funnel, UX and products to meet customers at their level and unlock significant growth and retention.
There are several established frameworks to help understand customer needs. One concept that can be helpful is the iceberg model of customer needs. This theory implies that a customer’s “position” — what they say – is only partially revealing of what they want. To truly understand, you need to go below the surface to tease out the underlying “why” behind what they are saying to understand the true must haves in a solution. Hint: How you solve a customer problem could take many forms especially with new tech. Ultimately this iceberg approach is better than some alternatives, but can still run into issues due to selection and confirmation biases of the marketing team to reflexively reject certain information that does fit their own preconceived notions.
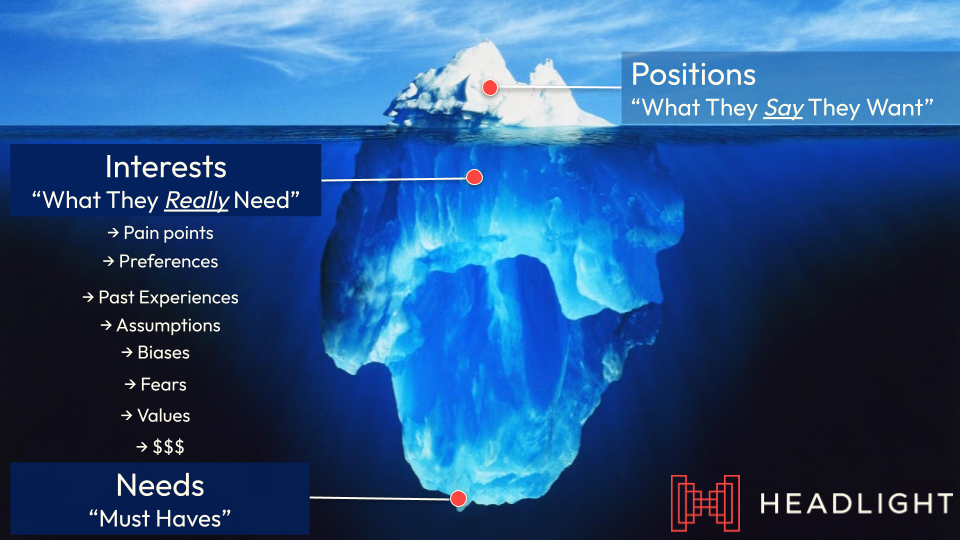
You may be thinking, ‘OK but we’re fine, we have customer personas’. Having personas is one thing, but when did you create those personas? According to a Nielsen Norman Group study, 46% of respondents update their customer profiles “infrequently” – only every 1-4 years. Many fundamental shifts in consumer behavior have occurred in the last few years, stemming from global pandemics, economic uncertainty, social unrest, privacy regulations and of course an emerging AI revolution. Needless to say, out-of-date personas mean you could be missing out on rapidly moving customer goal posts. Carving out and maintaining an edge in your market is greatly aided by a well-defined audience strategy driven by fresh competitive intelligence.
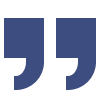
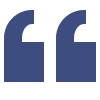
~Salesforce study, 2023
Enter ChatGPT and other Large Language Models
The rapid rise of large language models (LLMs) and ChatGPT has unleashed an incredibly powerful tool for collecting, synthesizing and understanding vast swaths of text and other data. Our focus here will be on ChatGPT, which with GPT-4 can process massive datasets using an 8,000 token context window. While the more advanced GPT-4 Turbo can process 128,000 token context window extremely quickly. This context window is in a sense ChatGPT’s “short term memory”. Note that tokens can be as short as a single character or as long as a single word so the actual word count may vary. So GPT-4’s context windows can be approximately 4,000-6,000 words.
What counts towards the context window total? Well, the context window includes both “the inputs” (i.e. questions, data and explained context) and “the output” (the summaries, analysis, answers, etc.). To put in well, context, this means within its context window, ChatGPT will factor in its prior analysis and what it said so you do not have to reexplain the same thing over again. In practical terms this means GPT-4 Turbo can process up to a 300 page book. If you exceed the context window the analysis can begin to drift and you may find a noisy result. So regardless of your model, you still need to guide with the right, relevant context.
Much testing has focused on how to apply AI capabilities into powerful new tools to solve problems. One advantage is ChatGPT’s “open-minded” approach to problem solving which can unlock hidden knowledge through more impartial review. However, if your underlying dataset includes bias so too will the output. In a strange way, ChatGPT’s approach is like a modern homage to the Socratic dialogues in a college philosophy class. As Socrates famously said, “All I know is that I know nothing” who obviously knew much, much more the AI also feigns ignorance before seeking insights. Examining an issue with an open mind is a helpful approach, where the interlocutor asks genuine questions and iterates prompts to achieve the right context, while the GPT assistant responds “impartially” to arrive at better solutions. Though there are still obvious downsides like hallucinations which we will discuss later. All these capabilities make LLMs a perfect tool for analyzing diverse customer reviews and feedback at scale, enabling savvy marketers to quickly spot trends, and adapt strategy to emergent needs. Finally, these models are improving at staggering speed, so their capabilities will have almost certainly improved by the time you’re reading this. Now that we’ve broken the ice, let’s get to the good stuff.
How can you take advantage of AI to gain actionable insights from your customer reviews? → Read Part 2: The Process
What are some of the pitfalls to avoid when using ChatGPT to analyze customer reviews? → Read Part 3: The Pitfalls & The Promise
Part 2: The Process of this series, we will walk you step-by-step through our process for using ChatGPT to generate fresh, actionable consumer intelligence that can be used to inform critical marketing and product decisions. Subscribe to stay informed, on how to incorporate this strategy and other trends relevant to your business.